1. 引言
在传染病领域,复杂网络理论可以用于构建传染病传播模型,揭示疾病在网络中的传播规律,通过考虑网络拓扑结构、节点间的联系方式以及传播动力学等因素,可以模拟传染病在人群中的传播路径和速度 [1] [2] [3] 。通过评估不同的疾病控制策略的有效性,模拟传染病在网络中的传播过程,可以优化控制措施,提高控制效率。利用复杂网络理论还可以对传染病的传播趋势进行预测和监测,及时发现传播风险区域和高风险人群,为疫情预警和应急响应提供科学依据。
双层网络模型被广泛应用于传染病的研究,为传染病研究提供了新的拓扑结构。一层为信息传播层,另一层为疾病传播层,两层网络一般存在着相互影响的因素,因为随着传染病的爆发,不仅仅只存在着病毒的传播,还伴随着一些信息或舆情,在双层网络中往往考虑了多个因素,例如信息层中的行为改变、负面信息、个体意识等等 [4] [5] [6] 。2013年,Granell等人首次建立了信息传播和疾病传播的双层网络,来探究以上两种传播过程的相互关系,并得到了流行病传播的阈值与双层网络拓扑结构变化的关系 [7] 。通过对信息传播中社交媒体影响的深入研究,2014年,Granell等人提出了更加成熟的疾病传播双层网络模型,并探讨了个体对疾病的认识与疾病传播之间的相互作用 [8] 。Kabir和Tanimoto提出了疾病和个体意识的双层传播模型SIR-UA,来探究个体意识对疾病传播的影响,并测试了在不同的网络拓扑结构下模型的效果 [9] 。在以上的双层网络研究中,两层节点均是一一对应的关系。而Guo等人建立了节点不是一一对应情况下的疾病传播和信息传播的双层网络,探讨了上层和下层网络节点间的对应率对疾病传播阈值的影响 [10] 。
我们引入了资源函数 [11] :
(1)
其中
表示感染者的最小恢复率,
表示感染者的最大恢复率,b代表资源量,I表示已被感染的人数。从式子中可以看出,函数
关于b是递增的函数,关于I是递减的函数,μ的数值在
和
之间。
2. UAU-SIS双层网络模型建立
本文构建的双层网络,如图1所示,下层是疾病传播物理层,上层是信息传播层,每一层网络的节点数量为N,且每一层网络的节点集合相同,信息传播层和疾病传播物理层的拓扑结构可以不相同。本文假设两层网络结构均为静态网络。上层网络节点有A (aware)和U(unaware)两种状态,A表示已知信息,U表示未知信息,下层网络节点有S和I两种状态,分别表示易感态和已感染态。因此网络中的节点理应一共有四种状态:US、AS、UI、AI,因为易感染态是肯定知晓信息的,所以双层网络中节点为UI状态的情况不存在。
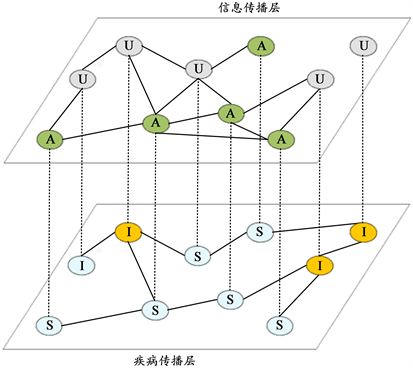
Figure 1. Schematic diagram of node status in two-layer network at a certain moment
图1. 某时刻双层网络节点状态示意图
设
和
分别是信息传播层和疾病传播层的邻接矩阵的元素。在t时刻,每个顶点i所处的状态概率分别为:
、
、
。
表示顶点i没有被任一邻点告知的概率,
表示网络中无信息顶点i没有被其任一邻点感染的概率,
表示网络中无信息顶点i没有被其任一邻点感染的概率,
表示网络中有信息健康节点患病的概率,设
表示网络中无信息健康节点患病的概率,且
。
用状态转移概率树可以很明了的看出节点的状态转移改变,因此构建了图2所示的状态转移概率树,概率树的树根表示网络中的节点在t时刻可能的三种状态,叶子表示网络中的节点在
时刻可能的状态。网络中节点的状态是在不断改变的,第一层箭头表示信息传播层节点状态改变,第二层箭头表示疾病物理传播层节点状态改变,并且在过渡的箭头上标注相应的概率。
若i节点为无信息节点,则与它相邻的有信息邻居的概率为
,则它被其中一个有信息邻居告知的概率为
,不被该邻居告知的概率为
,那么顶点i没有被任一邻点告知的概率为
,其数学表达式为:
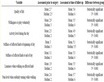
Table 1. Meaning of model parameter symbols
表1. 模型参数符号的意义
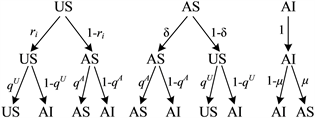
Figure 2. Transition probability tree of three possible states of nodes in a two-layer network
图2. 双层网络中的节点可能处于的三种状态的转移概率树
(3.2)
同理,有信息顶点i没有被其任一邻点感染的概率为
,无信息顶点i没有被其任一邻点感染的概率为
,其对应的数学表达式为:
(3.3)
(3.4)
其中,
,表示时刻t节点j处于有信息状态的概率。根据上述概念和等式,可以从t推导出
的方程等式,从而得到顶点的马尔可夫链方程。
得到等式:
(3.5)
同理可以得到:
(3.6)
以及:
(3.7)
3. 阈值求解
为了得到传染病传播的阈值,需要求出顶点状态的马尔可夫链进化方程的稳定解,在稳定状态下即
:
(3.8)
在求阈值过程中,假设
,所以得到:
(3.9)
并且在
时,
,
,将
、
和(3-9)带入到(3.5)~(3.7)中分别得到:
(3.10)
(3.11)
(3.12)
又因为
,
,所以式子(3.11)可以化简成为:
(3.13)
(3.12)可以化简成为:
(3.14)
又因为
,
,所以等式(3.14)可以进一步化简成为:
(3.15)
因为
的二阶项是
一阶项的高阶无穷小,所以可以忽略省去,因此得到:
(3.16)
(其中
是单位矩阵)
因此等式(3.16)的解等价于求矩阵的特征值,令一个矩阵为H,矩阵H的元素为
。所以疾病传播的阈值是满足(3.16)的
的最小值,因此当矩阵H的特征值取得最大值时,
的值能够取得最小值,所以临界点为:
(3.17)
所以当
时,疾病将无法传开,若
,则传染病将一直存在,并且最后处于非零的稳定状态。
又由:
(3.18)
得到:
(3.19)
又因为在稳定时刻(3.1)成立,带入上式中得到:
(3.20)
因此,将
带入
中,即可得到矩阵H的最大特征值,带入(3.17)可以得到阈值
。
4. 数值模拟
本节用MATLAB进行数值模拟,上下两层网络均构建有1000个节点,k = 6,连边概率p = 0.3的WS小世界网络。设定相同的初值
。
图3取参数λ = 0.015,δ = 0.01,βU = 0.15,βA = 0.05,μ0 = 0.05,b = 0.5 * N,在取不同的μ1数值下双层网络中三种状态的变化趋势。如图3中(a),(b)所示,在选取μ1 = 0.2和0.3的情况下,双层网络中AI状态始终存在且最后处于稳定状态,此时的阈值
和0.1396均小于βU = 0.15,因此疾病能够传播扩散。如图3中(c),(d)所示,在选取μ1 = 0.4和0.5的情况下,双层网络中AI状态的密度从增加到减少到为0,此时的阈值
和0.1915均大于βU = 0.15,因此疾病不能传播扩散,数值模拟结果验证了数学推导结果。
图4中取参数λ = 0.015,δ = 0.01,βU = 0.15,μ0 = 0.005,μ1 = 0.35,做出了在取不同的βA数值下双层网络中状态为AI的节点密度随着资源b的变化的拟合曲线,从图中可以看出随着资源b的增加,网络中状态为AI的节点密度不断减少到一定的数值,且随着获取信息健康节点患病的概率βA的增加,b在数值较小时网络中最终AI的节点密度差别不大,模型对βA的敏感度较小,但随着b的数值增加,网络中最终AI的节点密度变化较大,模型对βA的敏感度较大。并且随着b的增加,AI的拟合曲线的梯度逐渐减小,且在βA = 0.05当b大于0.8时AI的密度不发生明显下降趋势,因此资源的提升在一定的范围内具有显著的作用,且b的数值越小时发挥的作用越强。
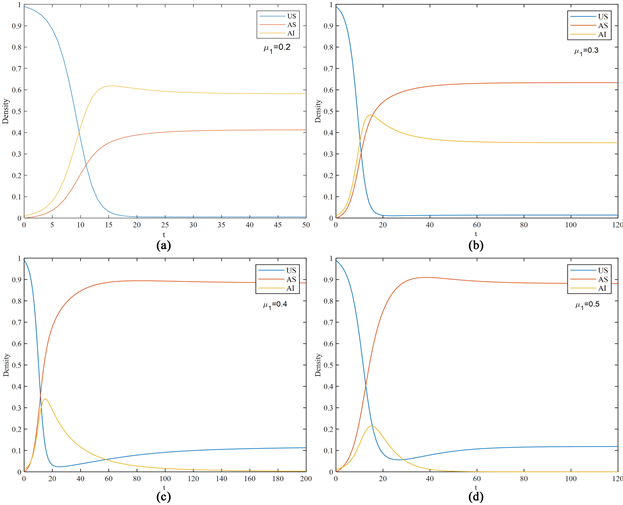
Figure 3. The variation trend of three states under different μ1 values
图3. 不同μ1数值下三种状态的变化趋势
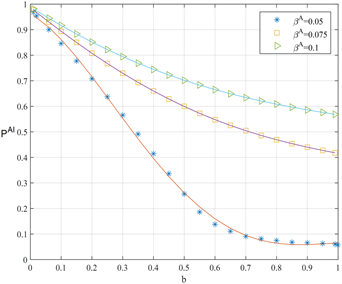
Figure 4. The influence of different values of βA on the node density of AI
图4. βA数值不同对AI的节点密度影响
5. 总结
本文提出了双层网络上的UAU-SIS模型,并且画出了该模型的概率转移树,通过概率转移树、运用MMCA方法,求出传染病传播理论阈值并进行了分析。通过数值模拟验证了理论阈值的准确性和仿真模型一定的正确性,得出以下结论:
(1) 资源在一定的区间内对传染病控制具有很好的效果,适当的医疗资源对遏止传染病的传播和传染病的控制有极好的效果,但是过多的医疗资源对控制疾病的效果不佳。
(2) 发现信息在疾病防控中具有重要的作用,资源和信息都影响着疾病传播的范围,不同的无信息健康节点患病的概率与有信息健康节点患病的概率比值将影响疾病是否爆发。
(3) 通过构建不同的网络结构,发现当信息和物理传播层的聚类系数都更高时疾病也能更快扩散和爆发,且感染密度更高,但当仅仅信息传播层的聚类系数高时,疾病得到了有效的控制,即信息在传播过程中发挥了遏制疾病的作用。
基金项目
国家社会科学基金青年项目——疫情散发背景下群体负向信息行为涌现机理与引导策略研究(No.22CGL050)。